Navigating the Privacy Paradox: Ethical Strategies for Responsible AI Use
- christopherstevens3
- Mar 6
- 8 min read
Updated: Mar 11
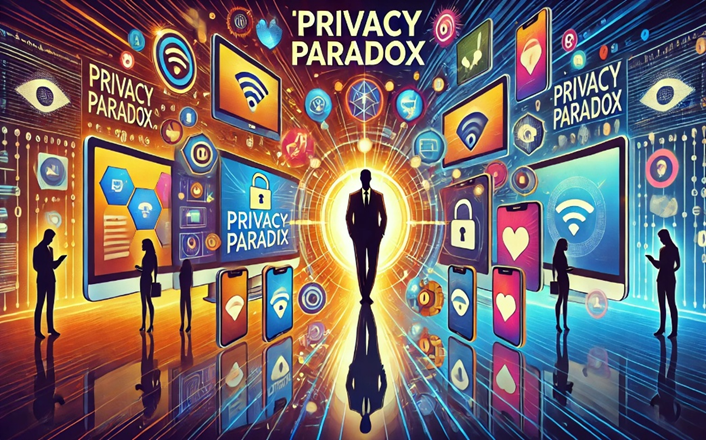
Introduction
Artificial Intelligence (AI) has rapidly transformed many aspects of modern life—from voice assistants like Siri and Alexa streamlining routine tasks—to sophisticated algorithms that personalize our online interactions. These advancements have seamlessly integrated into our daily lives, enhancing efficiency, convenience, and connectivity. Today, AI-enabled technologies recommend movies, suggest products, manage household routines, and even predict health risks with impressive accuracy. They are fundamentally reshaping user experiences across multiple domains. Alongside these remarkable conveniences, AI is also introducing significant ethical challenges notably concerning the handling, processing, and protection of personal data.
At the core of today's privacy challenges is the compelling and increasingly relevant phenomenon known as the "Privacy Paradox." Although people consistently express significant concerns about protecting their personal information, their actions frequently tell a different story. Why do users, fully aware of the risks involved, continue to willingly trade their privacy for convenience, personalized experiences, and AI-enabled services?
This paradox creates vulnerabilities that unethical entities can exploit, putting individual privacy at substantial risk. Addressing the "Privacy Paradox" requires moving beyond theoretical conversations and taking immediate, practical action. As artificial intelligence becomes more deeply embedded in our daily lives, understanding, and overcoming the privacy paradox is crucial to maintaining trust and safeguarding personal data in the digital era.
AI's Double-Edged Sword: Innovation and Intrusion
AI significantly benefits society, enhancing industries such as healthcare, retail, finance, and urban planning. For instance, precision medicine leverages AI algorithms to create personalized treatments tailored to genetic profiles, dramatically improving patient outcomes, reducing medical errors, and advancing early diagnosis and disease prevention. In retail, companies like Amazon utilize AI-powered systems to deliver highly targeted product recommendations, significantly enhancing customer satisfaction and driving increased sales and consumer loyalty. Financial institutions rely on AI for detecting fraud and automating complex trading strategies, improving security and financial efficiency. Urban planning benefits from AI through smart-city technologies that optimize traffic flows, energy consumption, and emergency responses, thereby improving public safety and quality of life.
However, these advancements carry notable risks. High-profile cases such as the Facebook-Cambridge Analytica scandal underscore how AI-enabled data analysis can be weaponized to manipulate public opinion, influence elections, and undermine democratic processes through unauthorized exploitation of personal data. The Equifax breach, exposing sensitive information of nearly 150 million people, vividly highlights the vulnerabilities inherent in large-scale data management and AI-driven systems. Such incidents emphasize the urgent need for stringent ethical standards, robust data protection measures, and transparent practices to mitigate these considerable risks effectively.
Decoding the Privacy Paradox
Why do users willingly sacrifice privacy despite their expressed concerns? The privacy paradox stems from deeply rooted psychological and behavioral tendencies. Users prioritize immediate rewards—such as convenience, personalized services, and instant gratification—over abstract, long-term privacy risks that feel distant or unlikely. Cognitive biases significantly influence these decisions. Optimism bias leads individuals to believe they are less susceptible to unethical behavior or misuse than others, while hyperbolic discounting pushes people to disproportionately value immediate conveniences, discounting future privacy implications.
Social factors also play a substantial role. Normative pressure, where users see others freely sharing information, reinforces the perception that extensive data sharing is socially acceptable or even necessary. Digital resignation, the belief that maintaining control over one's data is futile, further encourages passive acceptance of privacy risks. AI exacerbates these issues by creating increasingly personalized environments that are difficult to resist, subtly conditioning users to view data exchange as essential for digital participation. Recognizing and addressing these psychological and social dynamics is critical in developing effective solutions to the privacy paradox, ensuring that individuals can enjoy AI's benefits without unknowingly compromising their individual privacy.
Rethinking Consent in the AI Era
Traditional consent mechanisms involving lengthy, jargon-filled privacy policies fail to empower users effectively, as individuals rarely engage with or fully comprehend these extensive documents. This often results in uninformed consent, weakening personal data protection. Alternative consent approaches, however, offer significant potential for addressing these shortcomings. Dynamic consent, for instance, empowers users by allowing them real-time adjustments to their privacy settings and preferences, responding to evolving data uses and personal circumstances. Contextual integrity further enhances the relevance and clarity of consent by requiring that consent processes be clearly aligned with specific, context-dependent data usage scenarios.
Additionally, AI-enabled privacy assistants represent an emerging solution designed to further enhance user control. These intelligent agents provide personalized, intuitive privacy recommendations in real-time, simplifying complex privacy decisions by offering clear, actionable advice precisely when users need it. By bridging the gap between user intention and actual online behavior, these technologies help ensure that users can meaningfully and actively manage their digital privacy, mitigating the negative effects of the privacy paradox.
AI Ethics: Navigating Legal and Regulatory Challenges
Laws and regulations such as the European Union's General Data Protection Regulation, California’s Consumer Privacy Act as amended by the California Privacy Rights Act, and China's Personal Information Protection Law mark significant steps toward protecting individual privacy rights in the AI era. However, these legal and regulatory frameworks face considerable enforcement challenges due to rapid technological innovation and the inherent complexity of AI systems. For instance, regulatory agencies often struggle to keep pace with advancements in AI capabilities, which can quickly render legal frameworks obsolete or incomplete.
Moreover, ethical guidelines produced by academic institutions and industry groups aim to complement regulatory frameworks by promoting responsible AI usage. Yet, these ethical standards frequently lack universal consensus and can differ significantly across cultural, economic, and political context. Additionally, they contribute to confusion and inconsistent application globally. Consequently, multinational corporations and tech companies face difficulties navigating diverse ethical, legal, and regulatory landscapes. Addressing these challenges requires enhanced international cooperation, clearer global ethical standards, and agile legal and regulatory frameworks capable of adapting swiftly to ever-evolving AI technological developments.
Privacy-Enhancing Technologies: Tools for Ethical AI
Privacy-enhancing technologies (PETs) play crucial roles in addressing ethical challenges in AI and data management:
Data Masking and Tokenization: Techniques that replace sensitive data elements with fictional or meaningless values, widely implemented in industries processing large volumes of personal data, such as e-commerce, banking, and healthcare, to mitigate the risks associated with data exposure.
Differential Privacy: Employed by organizations like Apple and Google, differential privacy allows analysis of user data while adding noise to protect individual identities, effectively anonymizing data sets.
Federated Learning: Facilitates training AI models directly on user devices, eliminating the need to centrally collect sensitive personal data and reducing potential privacy breaches.
Homomorphic Encryption: Enables computation on encrypted data without requiring decryption, significantly enhancing privacy in fields such as healthcare, finance, and cloud computing.
Secure Multi-Party Computation: Enables multiple entities to collaboratively analyze data without revealing their individual data inputs, prominently used in financial services for secure analytics and fraud detection.
Synthetic Data: Offers artificially created data that preserves statistical accuracy without compromising privacy, allowing organizations to safely conduct AI training, testing, and research.
Collectively, these advanced PETs empower organizations to navigate ethical complexities, maintain compliance, and ensure that AI technologies respect individual privacy rights.
Charting a Practical Path Forward
Effectively addressing the privacy paradox requires a comprehensive and collaborative approach involving policymakers, technologists, businesses, academics, and society at large. Implementing "privacy by design"—embedding privacy into every stage of technology development—is essential. Adopting and integrating advanced PETs ensures privacy protections remain robust even as data processing capabilities evolve.
Enhancing transparency through clear, accessible communication about how personal data is used and providing users with meaningful choices regarding their data preferences is critical. Building accountable and transparent public-private partnerships can help establish clear standards, ethical guidelines, and oversight mechanisms.
Education and public awareness campaigns are also essential, empowering individuals to make informed choices and advocate for stronger privacy protections. Ultimately, fostering an ethical AI ecosystem depends on sustained, collective action across multiple sectors and stakeholders.
Crafting an Ethical AI Future
As AI becomes ever more intertwined with our daily lives, addressing the privacy paradox takes on critical urgency. This paradox is not just an academic or theoretical issue. It is a fundamental challenge shaping our society's relationship with AI technology, ethics, and privacy. Achieving meaningful progress requires more than technological innovation or regulatory enforcement alone; it necessitates a profound cultural shift toward recognizing privacy as a foundational human right rather than merely a convenience or optional consideration.
Policymakers must prioritize agile and responsive legal and regulatory frameworks that can quickly adapt to rapid technological advances while ensuring comprehensive data protection. Organizations and AI technology developers must embrace transparency not as a burden, but as an opportunity to build deeper trust and demonstrate ethical leadership. This includes clearly communicating data usage practices and actively engaging with consumers to help them understand and manage their digital privacy.
Educational institutions, media, and communities also have crucial roles to play. Raising awareness about privacy rights, data misuse risks, and effective ways to protect personal data is essential. Educational initiatives at all levels—from schools to corporate training—can foster greater AI literacy and privacy literacy, thus enabling individuals to recognize and mitigate privacy risks proactively.
Ultimately, crafting an ethical AI future requires a fundamental cultural shift in how we perceive and value privacy. When individuals are empowered with clear, meaningful choices about their data, and organizations commit to ethical responsibility and transparency, society can harness the transformative potential of AI without compromising fundamental human rights. The decisions we make today about privacy and AI ethics will profoundly shape not only our technological landscape, but also the very foundations of trust and autonomy in our increasingly digital world. They can help us better understand the “Privacy Paradox” and how it impacts our daily lives.
Reflective Questions for Readers:
Reflecting on your daily digital interactions, can you identify moments when you have knowingly traded your privacy for convenience? How might you approach these choices differently now after reading this article?
How might clearer, real-time explanations of personal data use influence your willingness to share personal data with AI-enabled products or services?
In your view, how can organizations ethically balance their personalized AI experiences with ethical and responsible data practices to build long-term trust with users?
What specific actions can you take personally or professionally to advocate for greater transparency and ethical accountability in AI-enabled personal data use?
References and Further Reading:
Apple. (n.d.). Differential privacy. https://www.apple.com/privacy/docs/
Bonta, R. (2024, March 13). California Consumer Privacy Act (CCPA).
Budin-Liosne, I., Teare, H.J.A., Kaye, J., Beck, S., Bentzen, H.B., Caenazzo, L., Collett, C.,
D ’Abramo, F., Felzmann, H., Finlay, H., Javaid, M.K., Jones, E., Katic, V., Simpson, A., &
Mascalzoni, D. (2017, January 25). Dynamic consent: A potential solution to some of
the challenges of modern biomedical research. BMC Medical Ethics, 18(4), 1-10.
Cadwalladr, C., & Graham-Harrison, E. (2018, March 17). Revealed: 50 million Facebook profiles harvested for Cambridge Analytica in major data breach. The Guardian. https://www.theguardian.com/news/2018/mar/17/cambridge-analytica-facebook-influence-us-election.
Creemers, R., & Webster, G. (2021, September 7). Translation: Personal information protection law of the peoples’ republic of China-effective Nov. 1, 2021. Stanford Umiversity-DigiChina. https://digichina.stanford.edu/work/translation-personal-information-protection-law-of-the-peoples-republic-of-china-effective-nov-1-2021/.
DataGuard Insights. (2025, January 23). The privacy paradox: Stay one step ahead of it. DataGuard. https://www.dataguard.com/blog/privacy-paradox/.
European Commission. (2025). Data protection. https://commission.europa.eu/law/law-topic/data-protection_en.
Federal Trade Commission. (2019, July 22). Equifax to pay $575 million as part of settlement with FTC, CFPB, and states related to 2017 data breach. https://www.ftc.gov/news-events/news/press-releases/2019/07/equifax-pay-575-million-part-settlement-ftc-cfpb-states-related-2017-data-breach.
GDPR: EU. (2025). Complete guide to GDPR compliance. https://gdpr.eu/.
Homomorphic Encryption Standardization. (2025). https://homomorphicencryption.org/.
IBM. (2023, January 31). What is synthetic data? https://www.ibm.com/ think/topics/synthetic-data.
Lindell, Y. (2020). Secure multi-party computation (MPC). Unbound Tech and Bar-Ilan University. https://eprint.iacr.org/2020/300.pdf.
Martineau, K. (2022, August 24). What is federated learning? IBM Research. https://research.ibm.com/blog/what-is-federated-learning.
Nissenbaum, H. (2009, August 19). Privacy in context: Technology, policy, and the integrity of social life. Stanford University Press. https://link.springer.com/article/10.1007/s10790-010-9251-z.
Psychology. (n.d.). Hyperbolic discounting. iResearchnet.com. https://psychology.iresearchnet.com/social-psychology/decision-making/hyperbolic-discounting/.
Sharot, T. (2012, February). The optimism bias [Video]. Ted Conferences. https://www.ted.com/talks/tali_sharot_the_optimism_bias/transcript.
Comments